Toward faster, better, cheaper ecosystem service assessments
“It’s just semantics” – Easy way to end a minor disagreement about what to call something
“Data collection and preparation takes up 60% of the time needed for environmental modeling” – apocryphal
Big data meets ecosystem services
Big data is everywhere these days – from Super Bowl ads for Google or Amazon cloud services to the real-time smartphone apps that navigate the least congested route across town. Big data has promised big things across the commercial and scientific worlds, and ecosystem services are no different. But what is big data, and why is it different from the status quo?
When we talk about big data, we’re not just talking about bigger, higher-resolution datasets than you could’ve dreamed of crunching on your desktop computer 10 years ago. At its heart big data meet the “3 V’s” criteria: they are high volume, high variety, and high velocity. Data aren’t just large – they’re highly diverse (watch your data preparation time shoot through the roof!) and coming in at much higher speed than in years past – and coming even bigger and faster in the future.
One of big data’s key added promises is to open the door for data-driven or inductive modeling, where computers learn patterns from huge volumes of data. This is a departure from classical, deductive hypothesis testing in science. Data-driven modeling will not make hypothesis testing obsolete, but promises a powerful complementary approach to advance scientific progress in the years ahead. Machine learning and different flavors of artificial intelligence are natural next steps to harness the rush of data across science, especially when trying to understand systems that are so complex that traditional hypothesis testing is likely to fail – which of course includes fields like ecosystem services.
As ecosystem service science begins to diversify its methods beyond early models that coupled satellite-derived remote sensing images with field data in ecological production functions, we’re beginning to see glimpses of next-generation ecosystem service assessments that integrate: (1) public satellite imagery archives and derived remote sensing products, from satellites like Landsat and Sentinel distributed through repositories like Google Earth Engine, (2) high-resolution commercial satellite imagery available from companies like Digital Globe or Planet Labs, (3) aerial images from both piloted aircraft and drones, (4) sensor networks, including the Internet of Things, and (5) crowdsourced, volunteered information, including both citizen science and social media. New data sources, faster computers, and creative new combinations of data and algorithms should allow an even greater variety of assessments as the field moves ahead.
In short, big data promises a bold new world for ecosystem services. That is, if we can get the key details right.
Data labeling & matching to link and reuse data and models: the missing piece
In an ideal world, that ever-growing pile of data would come in a ready-to-use form, with its content clearly identified and ready to plug into your favorite model (or better yet, a model that another scientist had independently made easily accessible to run through the web, and that you knew was appropriate for your time, place, and scientific question of interest).
This is the vision behind the FAIR Data Principles proposed by Mark Wilkinson and colleagues – that to maximize its value, scientific data should be Findable, Accessible, Interoperable, and Reusable. FAIR data are easy to find and access on the internet, and can operate in a transparent, plug-and-play fashion with your other scientific data and models. FAIR data are important because in a world of ever-growing data, you need confidence that data actually describe what you think they do. Unlike big-data analyses of internet searches or advertising clicks, scientific data are way too diverse (remember, high variety!) to link if you’re not confident that you’re connecting the right data in your models.
Back in 2001, Tim Berners-Lee, the scientist credited with inventing the Internet, published an article in Scientific American laying out a vision for the semantic web. Berners-Lee and his colleagues envisioned a world of data connected on the internet in the same way that web pages are: clearly and uniquely labeled and available for all to access. At its core, the semantic web is just a data labeling and matching system, which takes the ambiguity out of complex, diverse scientific big data and makes it more FAIR.
So what would we need to make our ecosystem services data and models fully FAIR? Two key pieces of infrastructure could help us bridge this gap – first, software tools to enable data and model linking and reuse – ideally tools that are open-source, user-friendly, and powerful, and second, the semantics, or data labeling and matching system, that would allow us to accurately label all the data inputs, outputs, and model components relevant to ecosystem services.
This second piece is especially challenging for an interdisciplinary field like ecosystem services – since it draws on diverse fields across the natural and social sciences. While it’s often possible to get a group of experts from a single scientific discipline like hydrology or genetics to sit down and agree to use the same terms to consistently describe data, the problem becomes vastly harder when we need very different disciplines to speak clearly and consistently to each other.
The Artificial Intelligence for Ecosystem Services (ARIES) project, in development since 2007, offers both the open-source software infrastructure and the data labeling and matching system to allow sharing, linking, and reuse of ecosystem service data and models on the cloud. ARIES is not a single model or even a collection of models, but a toolbox to manage and link existing data and models, adding flexibility, power, and speed to ecosystem service assessments.
ARIES has three key characteristics: it is (1) collaborative, enabling modelers to code models and share data on the cloud, (2) cloud-based, facilitating data and model sharing and use of high-performance computing, and (3) context-aware, letting users encode the time, place, and scientific questions their data and models can answer. As the system grows through the contributions of its users, an ever-expanding data and model library will enable users, assisted by artificial intelligence algorithms, to pick the best data and models to quantify ecosystem services and other aspects of socio-environmental systems in their own parts of the world.
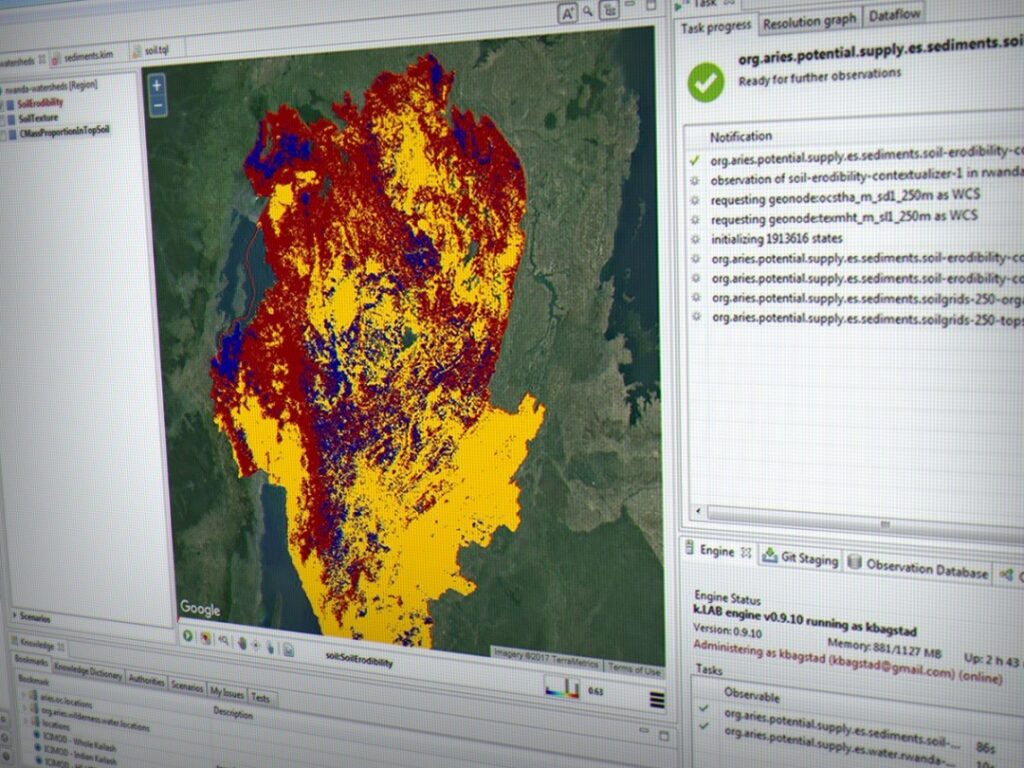
The road to faster, better, cheaper ecosystem service assessments?
Despite over a decade of intensive research, ecosystem service assessments still take too long to deliver to decision makers (with information delivered after the decision is made being of little use), and are often criticized as being too simplistic while at the same time too hard to use. Data reuse in ecosystem services has been minimal, making our assessments much more time consuming and sometimes less accurate than they need to be.
For example, the Ecosystem Service Partnership’s Visualization Tool is a potentially powerful interface for sharing the results of ecosystem service assessments. Yet the tool is rarely used (with 29 maps contributed from its launch in early 2015 through March 2018). While the data can be downloaded and reused, their annotation and distribution fall short of FAIR requirements that would let them easily and unambiguously be found and reused by people and automated computer systems.
Further, the Integrated Valuation of Ecosystem Services and Tradeoffs (InVEST) platform has been the most widely used ecosystem service modeling approach over the last decade, with many hundreds of users. Yet to date there has been little effort to share or reuse InVEST data, beyond a databases of publications and model parameters for its sediment and nutrient models.
This is not to criticize either of these efforts: both are valuable initiatives to provide credible, standardized tools and share results, improving transparency and reusability. However, they do embody today’s status quo of a “Tower of Babel” world: scientists speaking different languages that move the science forward in a sometimes haphazard way. Armed with an eye toward FAIR data, initiatives like these could move the ecosystem services community much farther ahead.
ARIES is thus far the only approach in the ecosystem services community to combine an open-source software infrastructure and the data labeling and matching system needed to enable assessments that are: (1) faster, with much less time needed to find, prepare, and align data and efficiently reuse existing models, (2) more flexible, being able to apply and link multiple modeling approaches and customize models to the time and place of focus, and (3) more accurate, with the ability to rapidly customize models using information from both scientists and local stakeholders – and to reuse that knowledge in similar places.
By consistently labeling and coding data and models, we can envision a more efficient and powerful landscape for the ecosystem services science of the near future. In support of the open-data movement, we could more seamlessly connect to scientific data repositories and other diverse data sources, allowing ecosystem services to move toward a longstanding goal of near-realtime assessment. Consistent data labeling and management would allow machine learning and artificial intelligence tools to be more widely tested and applied, letting us combine inductive and deductive science.
Finally, cloud-based models could be delivered through web interfaces that allow decision makers to access data and models, while technical modelers write and contribute the models to make such platforms possible. The approach doesn’t demand that modelers give up their preferred approaches, just that they label data and model elements consistently and in ways that models can be read within a larger modeling system. The growing ARIES model base serves as a knowledge base and prototype to grow such initiatives.
From a practical perspective, we can envision an ecosystem service assessment of the near future where scientists working in a new location run high-precision models in a matter of hours rather than weeks or months. This allows them to work more closely than before with stakeholders to improve and customize the model. At the end of the project, they share results in a way that lets other scientists more quickly and reliably use and build on the knowledge they have produced.
What separates us from this near-future world? Reliable and user-friendly software tools top the list; like other parts of the semantic web, ARIES’ software infrastructure has been assembled over time by a very small community and requires wider use, testing, and improvements, including the ability to reuse models written in popular programming languages. The related Integrated Modelling Partnership, launched in late 2017, offers one way for organizations to partner in development of key software infrastructure. Equipped with such software, scientists will be more easily able to code and contribute models and data to the semantic web for ecosystem services.
This could enable growth of a wider user community; the more people contribute data and models, the more powerful and flexible the system will become. A wider user community can also test and contribute to the semantics of ecosystem services: not just the names of the services themselves, but the data and model components needed to quantify them. The past year has seen important advances on both fronts: key updates to ARIES’ software, including completion of the long-planned ARIES web explorer, and the completion of a set of “global” ecosystem service models that can be run and customized anywhere in the world, making it easier for new users to access and run ARIES.
Yet ultimately, it will take the realization of the ecosystem services community to understand the value of collaborative data and model sharing on the semantic web – the FAIR, open-data future of ecosystem services on the semantic web, over the status quo, “Tower of Babel” approach to understanding ecosystem services. As the community comes to grips with the bottlenecks that limit the widespread use of our science, recognizing what the semantic web is and how it can help may allow development and application of 21st Century tools to overcome these bottlenecks and more sustainably manage our Earth’s resources.
About the author: Ken Bagstad is a research economist with the U.S. Geological Survey. He has contributed to the ARIES system’s design and data and model libraries since 2007.
Any use of trade, firm, or product names is for descriptive purposes only and does not imply endorsement by the U.S. Government.